Posts
Markov Text Generator
Perfect Inner Shadows
<feFlood x="-5%" y="0" height="100%" width="110%" flood-color="#222" result="COLOR-black" />
<feMerge result="source-on-black">
<feMergeNode in="shadow-bg" />
<feMergeNode in="SourceGraphic" />
</feMerge>
<feGaussianBlur in="source-on-black" stdDeviation="2" result="blur"/>
<feOffset in="blur" dx="0" dy="2" result="offset" />
<feComposite operator="in" in="offset" in2="SourceGraphic" />
Monitoring your life with Graphite
https://github.com/graphite-project/docker-graphite-statsd
docker run -d\
--name graphite\
--restart=always\
-p 8182:80\
-p 2003-2004:2003-2004\
-p 2023-2024:2023-2024\
-p 8125:8125/udp\
-p 8126:8126\
graphiteapp/graphite-statsd
http://docs.grafana.org/installation/docker/
docker run -d -p 3000:3000 grafana/grafana
$ sudo ip addr show docker0
4: docker0: <BROADCAST,MULTICAST,UP,LOWER_UP> mtu 1500 qdisc noqueue state UP group default
link/ether 02:42:3c:55:ac:f3 brd ff:ff:ff:ff:ff:ff
inet 172.17.0.1/16 scope global docker0
valid_lft forever preferred_lft forever
inet6 fe80::42:3cff:fe55:acf3/64 scope link
valid_lft forever preferred_lft forever
Heyzap Social App
Heyzap's social app allowed mobile gamers to share the games they play.
Games Feed
Quick access to your favorite games, and game discovery.
Stream
See what your friends are playing.
Profile

Web Audio Survey
WebAudio
Previously on Browser Audio
WebAudio ≅ <canvas> for Audio
<audio> ≅ <img>
Features
Compatability
Oscillators
Volume Control
Note Envelope
Tremolo (Amplitude Modulation)
Frequency Tremolo (Frequency Modulation)
Playback from file
Playback with timing
User interaction based timing
Capture microphone
Playback Rate
Delay & Fade Pitch Shift
PitchShiftLine
PositiveOscillatorNode
Combined
Gain A
Delay A
Gain B
Delay B
Frequency Filter
Doppler Effect
Convolution
Simple Reverb
Impulse Response Assets
Autocorrelation
Fourier Transform
Spectrogram
Switching to DuckDuckGo and Firefox
Returning to Firefox
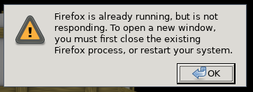
I began my descent to Chrome two years ago. At first, I only used Chrome while waiting for Firefox to load. Later I found Chrome made pages like Gmail and Grooveshark usable, and eventually stopped loading Firefox altogether.
Coming back to Firefox, things seem to have improved. Load times are comparable. The benchmark I ran still seems to indicate Chrome is roughly 30% faster, but pages feel equally responsive, and cnet reported Firefox to be faster.
Things I missed from Chrome (and fixed)
Tiny Tabs
Chrome allowed tabs to shrink to the size of favicons before scrolling. In Firefox, I was only able to fit around 10 tabs on the screen. Easy fix. Just install Custom Tab Width, and you can edit the minimum tab width from the add-ons pane.

If you have a more horizontal space than vertical Tree Style Tab might be an even better option.
Omnibar

Firefox separates the address and search bars. Coming from Chrome, that sounds insane. Use Omnibar to combine the two.
One problem left: Omnibar doesn't prefill a "?" in the address bar on [ctrl]-k
.
If you're an OSX user, Chrome didn't do this either,
but I really liked the confidence that [ctrl]-k
+ term would always search.
To bring back the question mark, install
Keyconfig.
Then hit [ctrl]-[shift]-F12
to bring up the Keyconfig pane,
and bind [ctrl]-k
to the following code:
gURLBar.value = "? "; gURLBar.focus();Make sure to unbind the default
[ctrl]-k
mapping.
Things I'm looking forward to
Lookahead search, "/"
I really love my keyboard shortcuts.
In Firefox, typing /downl[return]
will open a link titled "download" on the current page.
Firebug
Chrome Inspector is nice and all, but Firebug is clearly superior. I no longer recall why I hold this opinion. I'm looking forward to finding out.
Duck Duck Go
This is a bit more extreme, but the results are really quite good, and the interface is fantastic.
I assume you've tried DuckDuckGo out, but if not, do so. The results are a superset of Bing, which isn't quite Google, but it's usually okay. DuckDuckGo has picked up a lot of attention recently due to their privacy policy (they don't log queries), in contrast to most everyone else.
Again with the keyboard shortcuts
My favorite thing about DuckDuckGo so far is the vim style j/k navigation,
and [ctrl]-[return]
to open a link in a new tab in the background.
Or maybe the
Wolfram Alpha integration.
Or the instant Geoip lookup.
But the keyboard shortcuts mean I can search,
queue up promising results, and tab through them,
without reaching for the mouse, and using a very limited set of shortcuts.
Double checking with Google
The interface makes DuckDuckGo an excellent choice for a default search engine, but if I don't see the results I'm looking for, I want to double-check with Google to make sure I didn't miss anything. Adding !g to the end (or beginning) of a search term will send you to an encrypted google search.
Unfortunately, you either have to click the address bar, or hit h-[end]
before typing your !g-[return]
, which altogether is rather cumbersome.
I've uploaded a user script
for Greasemonkey
which binds "g" to focus the search bar, and append the bang!.
With this script, queue up all the promising looking links from your DuckDuckGo query,
then hit gg-[return]
to check if google has any better results
(it seldom does).
Giving to Google what is Google's
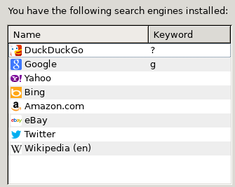
Some terms are clearly better handled by Google. Google is currently better with context-sensitive terms like showtimes, or local restraunts. I've heard it does better with long-tail searches, but I haven't found an example yet.
To provide instant google access,
I've bound [ctrl]-j
to prefix the address bar with a "g",
which is the keyword I've assigned to google in Firefox's
search engine preferences.
If you'd like to do the same,
install Keyconfig as above,
and bind [ctrl]-j
to
gURLBar.value = "g "; gURLBar.focus();
Conclusion
May you fare better.
Surfacing Interesting Content
Heyzap is The Largest Social Network for Mobile Gamers™, and as such, we get lots of user generated content. This is a collection algorithms we’ve been using to find the most interesting content. A lot of this is likely to be obvious, but I spent a bunch of time on the visualizations, so read it anyways.
Currently Popular
Do you need to know which songs your users are listening to? Which tags are trending on twitter? No need to break out a cron job, this algorithm will keep you up to date real-time.
What it Does
At Heyzap, we use this algorithm to display popular games. Each time a user plays a game, we cast a “vote” for that game. Each vote has a “score”, which decays with age. For our popular games, votes decay at a rate of 50% per week. To display the most popular games, simply add up all the scores for all the votes. Using this algorithm, a game played 20 times this week will be ranked “more popular” than a game played 30 times last week, and less popular than a game played 50 times last week.
To track trending hashtags, just replace “games” with “hashtags”, and cast a vote each time a tag appears. You could also use this algorithm to track word frequencies in newspapers, or which countries are visiting your site.
In the visualization above, votes are cast randomly at a set of items. The orange bars indicate the current “popularity score” of each item, and the red bars indicate the probabilistic rate at which each item should accrue new votes.
The longer the half-life, the slower the algorithm will respond to new votes. At the extreme ends, a half-life of zero would answer “Which post was most recently voted on?”, while a half-life of infinity would answer “Which post has the most votes?”.
How it works
A straightforward implementation might be:
- Each time a vote is cast, add 1 to the popularity score of the corresponding item.
- Once per day, divide all popularity scores by two.
You would probably actually want to multiply popularity scores by 0.97 each hour to minimize weird transients and make the decay more continuous.
However, I loathe cron jobs, and have an easier method.
- Each time a vote is cast, add 2**((now - epoch) / half_life) to the corresponding item.
As we only care about the rank of the popular items, the only difference between the outputs of the two implementations is that this one is perfectly continous, as opposed to the stuttering decay of the cron variation.
One drawback to the continuous implementation is float overflow. With a carefully chosen epoch, we can make use of a double-precision float’s 9 exponent bits and one sign bit to allow the algorithm to run for 2048 half-lives. If your half life is one day, you can run the algorithm for five years before needing to migrate the epoch.
Sidebar: Redis as an External Index
In all my examples, I’m using Redis as an external index. You could add a column and an index to your posts table, but it’s probably huge, which means that will be a pain. Additionally, since we only care about the most popular items, we can save memory by only indexing the top few thousand items.
If you’re not familiar with Redis, I’m using ZSETs. ZSETs are sorted sets. Half-array, half-dictionary. The value in the dictionary corresponds to the key’s relative “index” in the array. They have O(Log(N)) inserts, O(Log(N)) slices, and are indexed by double-preciesion foats, which make them perfect for this implementation.
Implementation
class PopularStream
STREAM_KEY = "popular_stream"
HALF_LIFE = 1.day.to_i
# 2.5 \* half_life (in days) years from now
EPOCH = Date.new(2015, 10, 1).to_i
def self.onVote(post)
# dict[post.id] += value
REDIS.zincrby(STREAM_KEY, post.id, 2 ** ((Time.now.to_i - EPOCH) / HALF_LIFE))
trim(STREAM_KEY, 10000)
end
def self.get(limit=20)
# arr.sort.reverse[0, limit]
REDIS.zrevrange(STREAM_KEY, 0, limit).map(&:to_i)
end
def self.trim(key, n)
# arr = arr[-n, n]
REDIS.zremrangebyrank(key, 0, -n) if rand < (2.to_f/n)
end
# run this in five years
# you could make EPOCH and STREAM_KEY dynamic
# to make this process easier. Otherwise migrate and deploy the new values
def self.migrate(new_key, new_epoch)
REDIS.zunionstore(new_key, [STREAM_KEY], :weights => [2 ** ((new_epoch - EPOCH) / half_life)])
end
end
Hot Stream
If the age of the post is more relevant than the age of the votes, we can simplify things considerably by treating all votes as though they were cast at the time the post was created. This is the algorithm used by Reddit’s front page.
What it does
If we start the decay for all votes on a post at the same time, we can simplify the formula for a posts score to:
post_creation_time / half_life + log2(votes + 1)
In the visualization below, votes are cast randomly on a series of posts. Each column represents the “hot” score of one post. The tallest column would be the #1 post on the “hot” page, the second tallest #2, and so on.
How it works
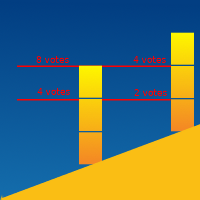
As I’ve tried to show in the picture above, adding a constant to log(votes) is the same as multiplying votes by a constant. log(c) + log(n) = log(n*c). So, each half-life we add to log(votes) doubles those votes power, giving us the same decay we had in the previous algorithm.
This means we don’t have to worry about overflows anymore!
Implementation
class HotStream
STREAM_KEY = "hot_stream"
# How long until a post with 100 votes is less interesting than one with 10 votes?
# Reddit uses 12 hours
TENTH_LIFE = 12.hours.to_f
# just to make it clear it's still the same algorithm
HALF_LIFE = TENTH_LIFE * Math.log(10) / Math.log(2)
def self.onVote(post)
# dict[post.id] = value
REDIS.zadd(STREAM_KEY, post.id, post.created_at.to_i / TENTH_LIFE + Math.log10(post.votes + 1))
trim(STREAM_KEY, 10000)
end
def self.get(limit = 20)
# arr.sort.reverse[0, limit]
REDIS.zrevrange(STREAM_KEY, 0, limit)
end
end
Drip Stream
This algorithm uses the same decay used in the hot steam, plus a threshold to create a digg-like, rate-limited, append-only stream.
What it does
Whenever a new post crosses the threshold, the threshold is incremented by the “drip period”, and the post is added to the drip stream. Since we’re constantly increasing the base score of each new post, a new post should be added to the stream once per drip period.
In the visualization below, votes are cast randomly on a series of posts. Each column represents the “hot” score of one post. The threshold is marked with a horizontal red line. As posts cross the threshold and are added to the drip stream, they are marked red.
Implementation
class DripStream
STREAM_KEY = "drip_stream"
THRESHOLD_KEY = "drip_stream_threshold"
# How long until a post with 100 votes is less interesting than one with 10 votes?
# Reddit uses 12 hours
TENTH_LIFE = 12.hours.to_f
# How often should a new story be pushed to the stream?
DRIP_PERIOD = 1.hour.to_f
def self.newVote(post)
return if REDIS.zscore(STREAM_KEY, post.id)
score = post.created_at.to_i / TENTH_LIFE + Math.log10(points))
threshold = (REDIS.get(THRESHOLD_KEY)||score).to_f + DRIP_PERIOD.to_f / TENTH_LIFE
if score > threshold
REDIS.set(THRESHOLD_KEY, threshold + DRIP_PERIOD.to_i / TENTH_LIFE)
# dict[post.id] = value
REDIS.zadd(STREAM_KEY, post.id, Time.now.to_i)
trim(STREAM_KEY, 10000)
end
end
def self.get(limit = 20)
# arr.sort.reverse[0, limit]
REDIS.zrevrange(STREAM_KEY, 0, limit).map(&:to_i)
end
end
Friends Stream
This creates a twitter-like stream of people/places/things you are following.
Isn’t that trivial?
Sure, usually. That’s why it’s at the end.
SELECT * FROM posts WHERE user_id IN (7,23,42,...) ORDER BY created_at LIMIT 20
Unfortunately, as you scale, IN queries get slow. Mongo pulls down 20 posts from each user, sorts them all by hand, then crops. When users follow thousands of other users, that gets slow. The SQL databases I tried at the time didn’t cut it either.
I no longer have the benchmarks. Don’t take my word for it. Just remember this is here if you start seeing thousand-entry IN queries in your slowlog.
How it works
The active ingredient is a ZSET of all users and their most recent post. That ZSET can be quickly intersected with the set of followed users, then sliced to create a list of recently active people you follow.
In this implementation, I’m using the actives list to union ZSETs containing each user’s stream. You could just as easily use the list to pair down the arguments to your IN query.
Implementation
class FriendsStream
USER_STREAM_KEY = lambda{|user_id| "user_stream_#{user_id}"}
USER_FRIENDS_KEY = lambda{|user_id| "user_friends_#{user_id}"}
USER_ACTIVE_FRIENDS_KEY = lambda{|user_id| "user_active_friends_#{user_id}"}
FRIENDS_STREAM_KEY = lambda{|user_id| "friend_stream_#{user_id}"}
ACTIVE_USERS_KEY = "active_users"
def self.follow(user, to_follow)
REDIS.sadd(USER_FRIENDS_KEY[user.id], to_follow.id)
end
def self.push(post)
REDIS.zadd(USER_STREAM_KEY[post.user_id], post.id, post.created_at.to_i)
trim(USER_STREAM_KEY[post.user_id], 40)
REDIS.zadd(ACTIVE_USERS_KEY, post.user_id, post.created_at.to_i)
trim(ACTIVE_USERS_KEY, 10000)
end
def self.get(user, limit=20)
REDIS.zinterstore(USER_ACTIVE_FRIENDS_KEY[user.id], [ACTIVE_USERS_KEY, USER_FRIENDS_KEY[user.id]])
active_friends = REDIS.zrevrangebyscore(USER_ACTIVE_FRIENDS_KEY[user.id], 0, limit)
REDIS.zunionstore(FRIENDS_STREAM_KEY[user.id], active_friends.map(&USER_STREAM_KEY))
REDIS.zrevrange(FRIENDS_STREAM_KEY[user.id], 0, limit).map(&:to_i)
end
end
Bring back the old gmail
Update: Sorry guys, the game is up. Gmail has now moved 100% to the new look
Run as a bookmarklet
Drag it to your bookmarks toolbar, refresh gmail, and click
you really should look over the source first
Run in the console
Refresh gmail and paste the code below into the console
<%= inline(@js) %>
Magic Text View
Featuring:
- Crisp Outer Shadows as many as you like!
- Inner Shdows again, hundreds if you so desire
- Outlines just one for now, add more and send a pull request for extra credit
- Text Backgrounds "Fire" made of fire!
- Custom Font Faces because honestly, droid-sans?

Force Feed - Speed Reading Bookmarklet
Force Feed teaches you to read faster by forcing you to read faster. Instead of scanning back and forth as you move down the page, Force Feed flashes the text word-by-word in a box fixed to the top of your screen. It’s a little like a flip-book for text.
Keeping pace with the flashing text requires more focus than reading slowly, and it’s pretty exhausting at first. However, like most things, it does seem to get easier with practice.
To get started, drag the Force Feed link to your bookmarks toolbar, and click your new bookmarklet to bring up the Force Feed toolbar. Then click on any sentence on the page to begin reading.
Try it out on some story somewhere else!
Keyboard Shortcuts
J/K : skip ahead / skip backwards
H/L : speed up / slow down
P : pause / resume